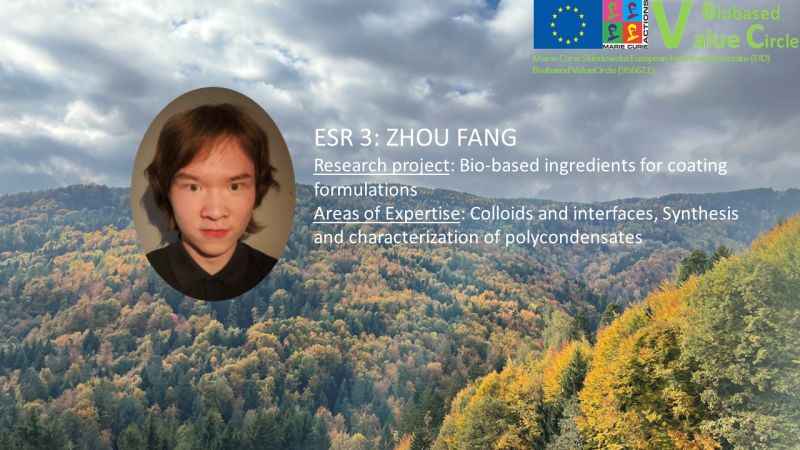
Abstract
Galactaric acid is a molecule derived from sugar beet pulp, a waste stream from sugar production. Due to the presence of secondary OH groups that might trigger branching or cross-linking, it is risky to directly use this molecule for polyester synthesis. The goal of this thesis is to extend the value chain of galactaric acid by exploring the possibility of transferring the acetal-protected from (GalX) and catalytic converted form (2,5-Furandicarboxylic acid, FDCA) of galactaric acid as building blocks into degradable thermosetting polyester resins. It was found that the cross-linking can be carried out by two means including initiator-free UV and thermal treatment. Cured thermosetting polyesters were proved to be degradable after the end of their service lives serving as coating and adhesive resins thanks to the labile linkages including acetals and esters. Moreover, to accelerate the development of bio-based polyesters, machine learning models were developed for predicting the glass transition temperature (Tg) of new polyesters derived from FDCA with high accuracy. Overall, this research proved that it is feasible to put the derivatives of galactaric acid into applications and the synthetic approach as well as the models developed in this thesis may contribute to the circular material transformation.
Publications for Communication and Dissemination
Action plans | Target actors/audience | Means | Timing |
AMIBM Scientific Colloquium | Academic staff and students at Maastricht University | Presentation | January, 2022 |
Circular Biobased Performance Materials Symposium, Wageningen | Academic; all fields of biobased sources for new chemicals and materials and circularity of products | Participator | June, 2022 |
AMIBM Scientific Colloquium | Academic staff and students at Maastricht University | Presentation | January, 2023 |
Dutch polymer days | Academic and industry; all fields of polymer | Presentation | April, 2023 |
LinkedIn Biobased Value Circle | Academic/Industry and general public; wide range of professionals | Promotion | November 2023 |
Biobased Value Circle Symposium | Academic and industry; all fields of polymer | Presentation and poster | May, 2024 |
Belgian Polymer Group meeting | Academic and industry; all fields of polymer | Poster | May, 2024 |
Prague Meeting on Macromolecules (PMM). | Academic and industry; all fields of polymer | Presentation | June 2024 |
Protection of the acquired intellectual property (patents applications) etc.
Not applicable.
Impact on science and/or technology
- The new synthetic procedure for monomers reported in the manuscript would help researchers who aim to create cyclic acetals from thermal-sensitive aldehydes/ketones and secondary hydroxyl groups with relatively low reactivity more efficiently
- The structure-property relation study for polymers could be a good reference for developing (bio-based) synthetic polymer resins with targeted properties
- This work will be the first research that reports a curing method using the UV-induced [2+2] cycloaddition for coating applications with the advantage of initiator-free nature
- This strategy of creating polymer networks based on diethyl galactarate-derived acetals can provide new insights for making degradable thermosets, which would be beneficial for solving environmental problems brought by the conventional long-standing polymer thermosets
- The models developed show that specific and consistent dataset can achieve accurate prediction even with a small dataset for training. Other researchers or product developers may apply similar approaches for other polymer properties of interest so that their polymer development can be facilitated.
Impact on innovation (companies)
Similar as the scientific/technological community, the companies may acquire insights in developing bio-based (especially sugar-based) degradable thermosetting materials for their innovative products thanks to the industrial nature of the doctorate program.
Impact on society
Environmental Impact:
- Reduced Plastic Pollution: Bio-based and degradable polymers, especially thermosets that generally cannot be re-processed can significantly reduce plastic pollution in landfills and oceans. This benefits everyone as it creates a cleaner environment, healthier ecosystems, and potentially reduces microplastics entering the food chain.
- Sustainable Material Production: Bio-based polymers can be derived from renewable resources, reducing reliance on fossil fuels and promoting a more sustainable circular economy. This benefits policymakers, businesses, and the world as a whole by mitigating climate change and promoting resource conservation. This project may help to reach the EU Green Deal targets by 2050 since we combine bio-based raw materials with degradability of thermoset networks.
Societal Impact:
- Improved Product Safety: Considering the toxicity of initiators, the initiator-free routes for curing explored in this research can be used to create safer products, especially for children’s toys or medical applications. This benefits consumers and promotes responsible manufacturing.
- Reduced Reliance on Landfills: As these polymers degrade, they reduce the amount of waste going to landfills, extending their lifespan and reducing the need for new landfills. This benefits local communities and policymakers.
Additionally, machine-learning assisted polymer development can:
- Accelerate Innovation: By automating tasks and predictions, machine learning can speed up the discovery and development of new bio-based polymers with desired properties. This benefits businesses and researchers by bringing these solutions to market faster.
- Reduce Development Costs: Machine learning can optimize material combinations and production processes, potentially reducing the cost of developing and producing new polymers. This benefits businesses and consumers by making these materials more affordable.
Visual Summary – Poster
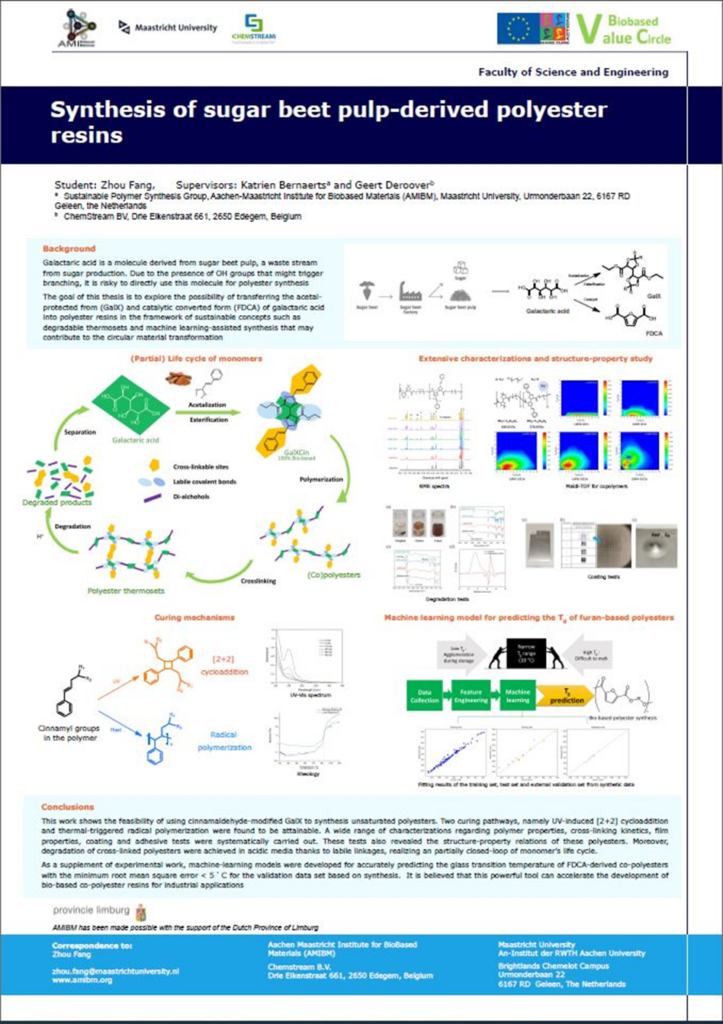